Are Low Code platforms helping solve the skills crisis?
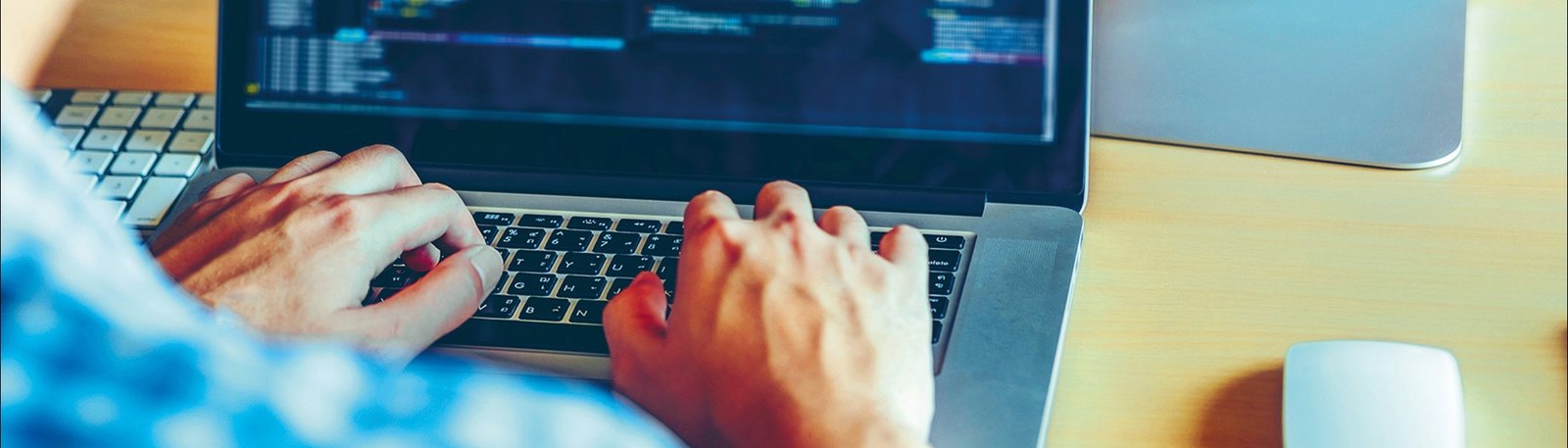
Platforms which allow lay users to create apps, process data and even perform machine learning with minimal or no code are on the rise. Can they plug the digital skills gap?
We need more experts – a lot more experts. A 2021 government report, Quantifying the UK Data Skills Gap, found a shortfall of 178,000 to 234,000 individuals with data competency in the UK alone. It also found that nearly half (48 per cent) of businesses are recruiting for data roles, with 46 per cent struggling with recruitment. Digital skills – from data analytics to cyber security – are in short supply, with demand coming from far more than just the tech sector. In fact, Microsoft estimates that 77 per cent of jobs will call for technical skills in the next decade.
This is not just a problem in the UK; in no part of the world can there be said to be an abundance of software engineers. The US Bureau of Labor Statistics estimates the global shortage of software engineers could reach 85.2 million by 2030.
Professor Rachid Hourizi, director of the Institute of Coding, attributes the digital skills gap to continued rapid advances in tech while education trails far behind: “Fundamentally, this is an area in which the world is changing very, very rapidly and therefore the challenge to keep up is exacerbated. It’s not an ancient or medieval industry that hasn’t changed much; in a year’s time the digital sector will have changed again and will have different requirements.”
Prompted in large part by the digital skills gap, a certain type of platform is on the rise. These platforms – which are mostly available as ‘platform as a service’ (PaaS) solutions – aim to make app development and other technical activities accessible to lay users. In essence, they seek to make a reality from GitHub CEO Chris Wanstrath’s confident assertion that: “The future of coding is no coding at all.”
Low-code and no-code (LCNC) development platforms tend to have intuitive interfaces which enable the user to build applications using visual building blocks – such as point-and-click and pull-down menus – rather than from scratch. No-code platforms require no knowledge of programming languages at all, while low-code platforms allow users to add their own code to auto-generated code. This lowers the barrier to app development to include ‘citizen developers’ who may become adept at designing apps while knowing no programming languages at all.
Big players in this space include Microsoft (Power Platform and PowerApps), Salesforce (Lightning, Force.com and others), and Siemens (Mendix). One of the most popular uses of LCNC development platforms is for designing automated workflows that reach into multiple systems to perform simple administrative tasks, known as robotic process automation. Other common uses include virtual chatbots for company websites, case management systems, performance appraisal tools for HR and reservation management for restaurants. LCNC platforms offer more than app development, however – there is a growing number of platforms which provide tools for lay users to perform visual analytics, machine learning and other advanced technical processes.
LCNC platforms offer an alternative to businesses, which have traditionally been made to choose between ready-made third-party applications and bespoke applications designed and built for their needs by skilled developers (‘pro-code’) at great cost. LCNC allows businesses to access tailored applications significantly more cheaply and quickly than before. Research from Forrester indicates that low-code development platforms could help businesses complete projects as quickly as 20 times faster.
One might expect LCNC platforms to be eyed with a certain amount of hesitancy and suspicion, and undeniably there are some concerns among IT professionals. For instance, LCNC platforms can give rise to ‘shadow’ applications created independently of a company’s IT department – and, thanks to their opacity, it can be tricky to ensure they are kept secure and compliant in the long term. However, these platforms have been broadly met with relief and enthusiasm as a means of providing technical services at a time when there are not nearly enough engineers to meet demand; Salesforce said that 92 per cent of experienced IT leaders are comfortable with their businesses using low-code tools.
LCNC platforms saw rapid growth during the pandemic in the face of increased demand for bespoke digital services and the widespread physical dispersal of organisations. KPMG found that, since the outbreak of Covid-19, the proportion of executives identifying low-code development platforms as their most important automation investment almost tripled from 10 to 26 per cent. A Gartner report from 2021, meanwhile, predicted that LCNC platforms would be used in more than 65 per cent of app development projects by 2024.
By auto-generating code, LCNC platforms cast doubt on a popular assertion regarding digital skills. It is often said that the ability to write code should be considered a skill as basic and essential as reading, writing and arithmetic.
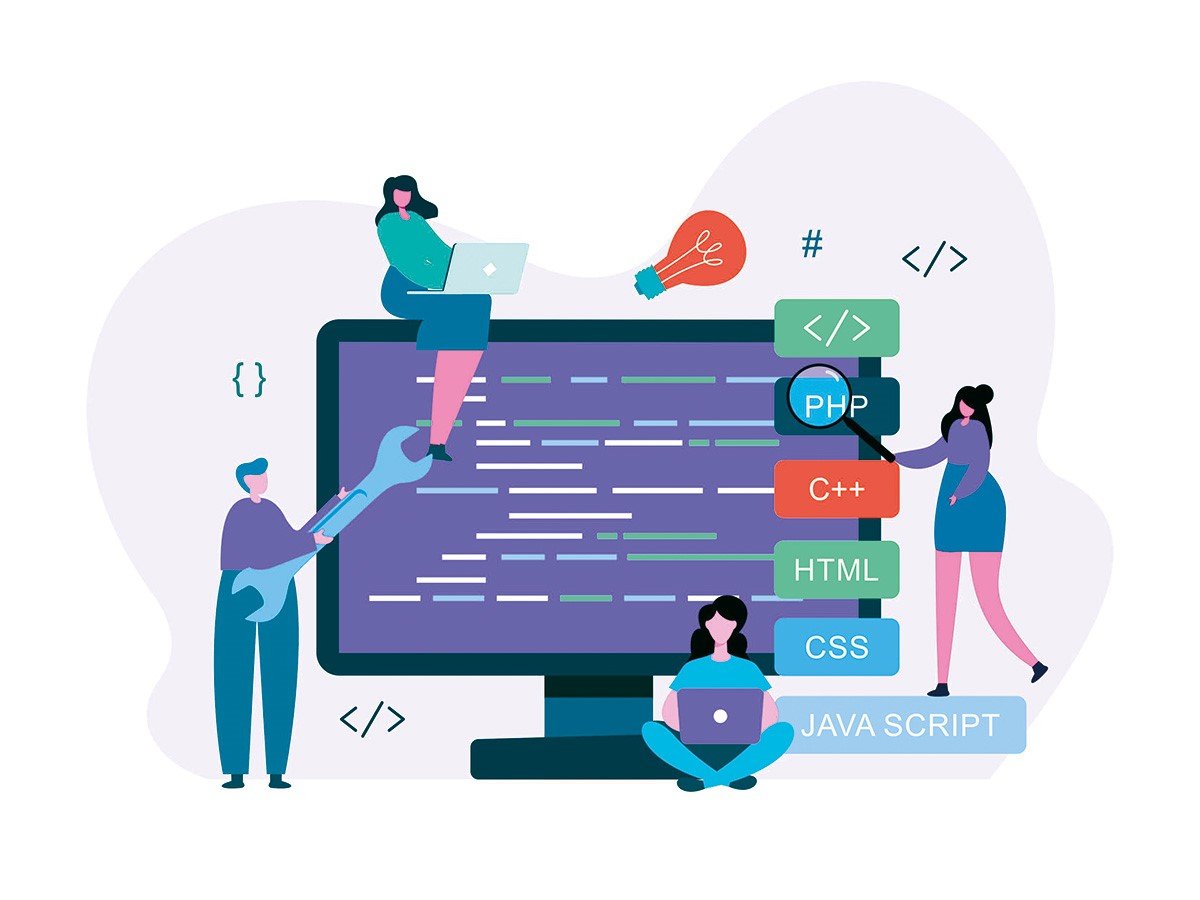
There is a counter-argument to be made that it is unnecessary for everyone to learn to use programming languages – in fact, this might be a distraction from other areas of expertise they might have far more aptitude for. Is the expectation that everyone should learn to write code as impractical as the expectation that everyone should learn to solder a circuit board?
“Not all humans can – or should – be writing in code. Python doesn’t make a person a remarkable data analyst, and so it should not be a required skill for data analytics,” says Libby Duane Adams, co-founder and chief advocacy officer at Alteryx, which provides LCNC data analytics software. “A code-free data analytics platform opens the way for great problem-solvers: the people with the business context needed to solve their own questions [...] having people with expert domain knowledge, and upskilling them with data analytics tools means they can work more closely with the data science team and, in many cases, solve their own problems at their own speed.
“The only reason that analytics has stayed in the remit of data scientists is because – until recently – there was no other option for non-coding experts to contribute,” Duane Adams continues. “We’re at a really exciting time for analytics today, because the data that people need is everywhere, and more people want to unlock the valuable nuggets of insight hidden in that data.”
It is neither essential nor realistic for everyone using these tools – or any other technologies for that matter – to be capable of building them from first principles. So, how much technical understanding is essential for users of LCNC platforms? Given the diversity of tools and use cases, there is no answer more precise than ‘more than none’.
For certain areas, however, we might expect a higher level of understanding. Machine-learning applications present ethical problems when results emerging from a black box are used in real-world decision-making, often entrenching existing inequalities and unconscious bias. Dr Matthew Forshaw, a data scientist at Newcastle University and senior advisor for skills at the Alan Turing Institute, says that research has revealed a widespread lack of consideration for ethics in data science master’s degree programmes. This could be worsened with this rush to upskill people as quickly as possible to fill the digital skills gap: “These [LCNC] tools lower the barrier to entry quite significantly, but I suppose what they bring into sharp focus is: what sort of checks and balances do we need in place to know that we’re not introducing algorithmic bias? We have a challenge there, where there can be unintended consequences if people are not considering the assumptions that particular models are making.”
As LCNC platforms grow, there must be a corresponding growth in understanding of how they work – not only via more people choosing to study technical degrees, but also learning through apprenticeships, adult courses, and self-teaching with open-source resources. If these tools are relied upon by people who do not understand them, they cannot be considered truly democratising – instead, they could have the effect of concentrating power and knowledge in the hands of a few platform controllers.
“If I was in a position where I was hiring a data scientist, [I would care about] responsible use of the black box. If you’re delivering business value, I don’t care if you’ve coded this from scratch or used a tool; what I care about is that you know what it’s doing,” says Forshaw. “It doesn’t need to be the conventional university education that gives people that [knowledge].”
LCNC platforms seek to fill the digital skills gap and, in many ways, they are successful. They are already easing burdens on software engineers by allowing citizen developers and automation to take over elements of their work. By allowing adults to upskill quickly, they also present a solution to one of the biggest challenges, which is that the digital skills gap cannot be filled simply by expanding school- and university-level education (80 per cent of the workforce of 2030 is already employed).
However, LCNC platforms cannot provide for all app development needs, being fundamentally limited in scope for customisation. For most large-scale and mission-critical projects, businesses will still turn to software engineers for bespoke solutions built from scratch.
There is also the fact that – as is the case with robotic automation – highly skilled people are not only required to design, build and maintain these platforms, but also to use, integrate, monitor and scale them. These latter needs are likely to give rise to demand for distinct new sets of skills, such as in human-computer interaction.
“We firmly believe we can’t go ahead with digital skills without having interpersonal skills,” says Rehan Haque, founder and CEO of AI-powered talent marketplace metatalent.ai. “We have to write code to communicate with robots, we need to have a human element there.”
The rise of LCNC platforms presents many opportunities for businesses, but – such is the size of the digital skills gap – they do not begin to negate the need for a massive increase in software engineers, data analysts, machine learning engineers and other individuals with advanced digital skills. “It is very clear that we need more experts: people to write systems, people to check systems, people to integrate systems,” says the Institute of Coding’s Hourizi. “We need more people working in data science and AI who are educated to a very high level, whether that’s bachelor’s degree, master’s degree or PhD, we have far fewer of them than we need.”